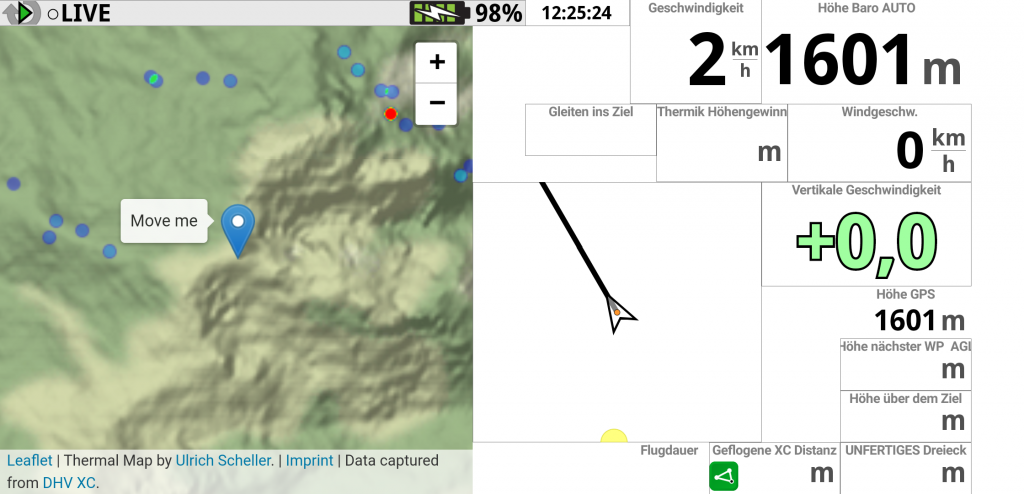
See the Thermal Map while flying
Many pilots asked me if it is possible to use the Thermal Map while flying. And indeed, this was the initial idea of the whole project. But it is hard to debug when you need
Many pilots asked me if it is possible to use the Thermal Map while flying. And indeed, this was the initial idea of the whole project. But it is hard to debug when you need
The Thermal Map has gained traction in the paragliding community. The well-known German blog Lu-Glidz has written an article explaining how to use it. Usage of the website has peaked after the article was published
This post is a followup to the last one about Paragliding data gems. We have collected lots of flights and their GPS location data. From this, several million thermals were extracted and shown on a
Paragliding is my beloved hobby and besides offering stunning views and perfect days outside, it also provides a huge amount of flight data to process and play around with. Sites like xc.dhv.de, XContest contain millions
Python is a great language for the on-demand style of Lambda, where startup time matters. In terms of execution speed, there are better choices available. Where computational performance matters one improvement is to use Pypy, the Python interpreter
Serverless computing is a cloud-computing execution model in which the cloud provider dynamically manages the allocation of machine resources. At my current project, I had the freedom to create a new service a service from
Android developers vs. web One great advantage of native apps over web apps is that they don’t depend on an online connection to start. Therefore the startup is fast and you can use them in
As a native apps developer since 2008, I have seen time and time again the wish to develop everything with one toolset. Most often, the toolset of choice is the web, or HTML, CSS and
My webhoster 1blu has finally added the possibility to get a free LetsEncrypt SSL certificate for this website. So www.ulrich-scheller.de is now available via HTTPS. Let’s Encrypt With LetsEncrypt getting an SSL certificate is free. There
Virtual Reality is a hot topic these days. A few weeks ago I had the opportunity to test an Oculus Rift with Touch Controllers. PlayStation VR and HTC Vive have also been released lately. Android Developers like