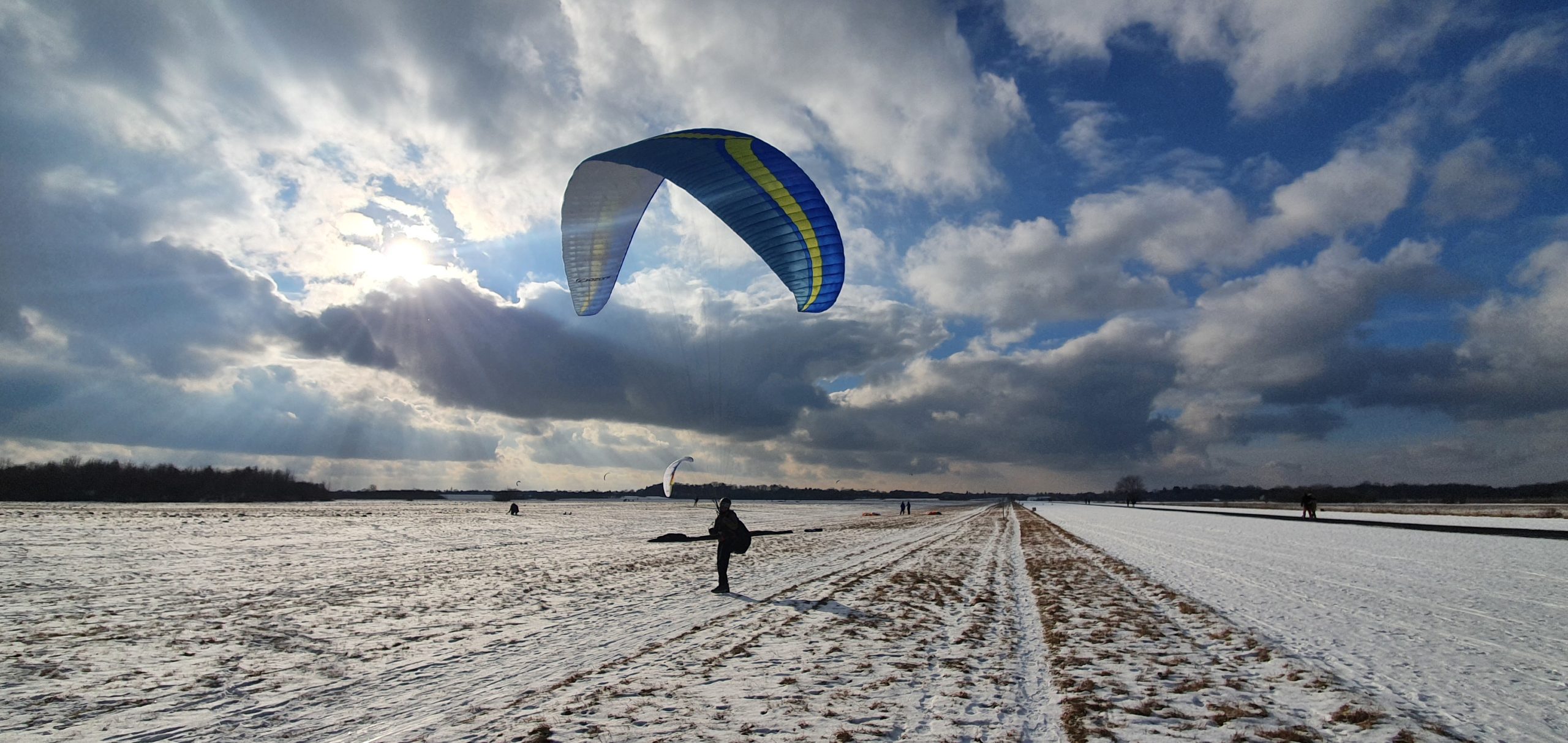
Calculate wind from thermals
This post is a followup to the last one about Paragliding data gems. We have collected lots of flights and their GPS location data. From this, several million thermals were extracted and shown on a
This post is a followup to the last one about Paragliding data gems. We have collected lots of flights and their GPS location data. From this, several million thermals were extracted and shown on a
Paragliding is my beloved hobby and besides offering stunning views and perfect days outside, it also provides a huge amount of flight data to process and play around with. Sites like xc.dhv.de, XContest contain millions
Python is a great language for the on-demand style of Lambda, where startup time matters. In terms of execution speed, there are better choices available. Where computational performance matters one improvement is to use Pypy, the Python interpreter
Some of the developers at match2blue are creating a text-interest-matcher. Leaving buzzword bingo aside, that means the software calculates whether a text is interesting based on users’ interests. So basically you, as a user, have