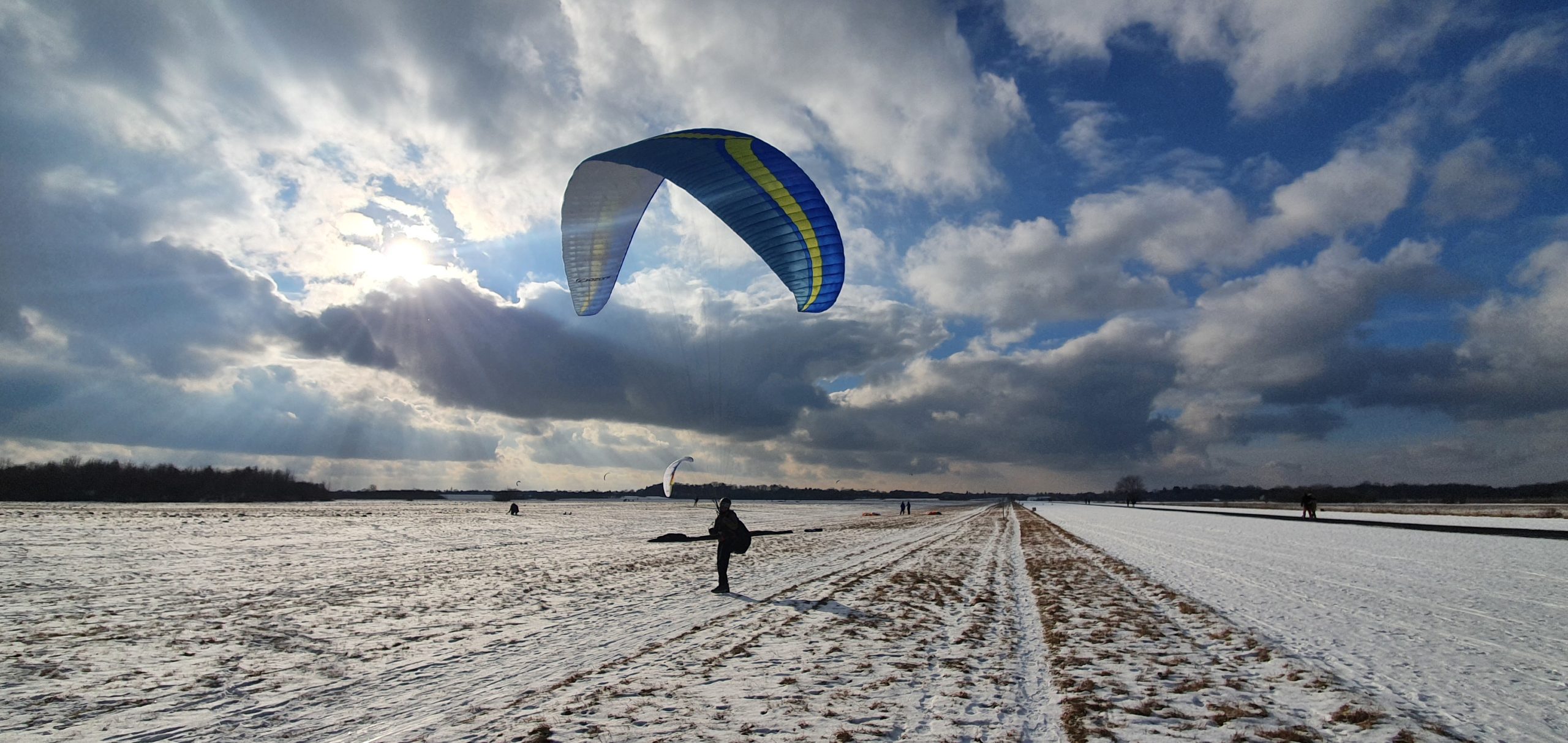
Calculate wind from thermals
This post is a followup to the last one about Paragliding data gems. We have collected lots of flights and their GPS location data. From this, several million thermals were extracted and shown on a
This post is a followup to the last one about Paragliding data gems. We have collected lots of flights and their GPS location data. From this, several million thermals were extracted and shown on a
Paragliding is my beloved hobby and besides offering stunning views and perfect days outside, it also provides a huge amount of flight data to process and play around with. Sites like xc.dhv.de, XContest contain millions
Nowadays, every software development team should have a continuous integration server like Jenkins. This is as true for Android developers as for any other platform. It makes sure the current source code compiles and all the
One thing that helps me a lot during my everyday routine is having shortcuts for every simple action I perform. For example uninstalling a specific Android app opening the current XCode project opening a ‘tail